Introduction to Data Storytelling for Sports
This is the first newsletter in an email course focused on Data, AI and Design: Data Storytelling for Sports.
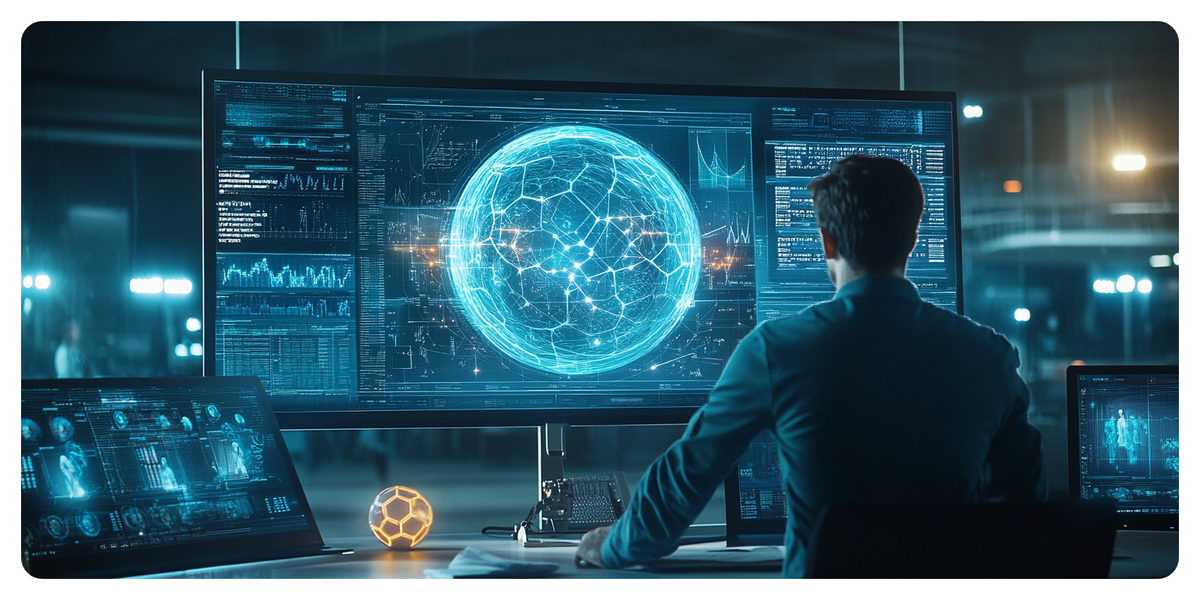
At a Glance
- About the Data Storytelling for Sports Series
- What is Data Storytelling?
- What is Data Storytelling for Sports?
- Sample Data Story: When do NHL Superstars Peak?
About the Data Storytelling Email Course
An often-overlooked aspect of sports and data analysis projects is data storytelling. It's one thing to crunch the numbers, but it's yet another to use that data to tell a story. It's where science meets art.
Over the next ten weeks, we're going to focus on data storytelling for sports series – an email course if you will. In the series, we'll cover the basics of data storytelling, how to explore your data to find the story, translating a data analysis into a narrative, how to leverage AI in data storytelling, key design principles and resources, different modalities in data storytelling, and how to produce your content.
What you'll learn is that while there can a lot of time bound up in analyzing the data, you should be spending equal amounts of time preparing the narrative using that data. And this is not just about removing "chart junk"; it's about defining your audience, choosing a content modality, creating a hook for and a narrative around well-presented data, and it's about making it interesting – all for something that could be consumed in the moment.
By the end of the series, you'll have learned the fundamentals of data storytelling and how you can apply these lessons and best practices in your own everyday use of data in your choice of narrative. Whether it's sports journalism, presenting sports analytics or analyses to management, creating social content or something else, you'll take away something valuable from the series.
In this newsletter, we'll cover what is data storytelling, where data storytelling fits within the creator economy, the role of AI in data storytelling, how data storytelling for sports is different, and we'll introduce the example data story that will guide the hands-on portion of our course.
Feeling lazy? Check out the quick-hit video for this newsletter below.
What is Data Storytelling?
In today's digital landscape, data is everywhere. But raw data alone isn't enough to make an impact. To truly resonate with an audience, data must be transformed into a compelling narrative—this is the essence of data storytelling. Data storytelling is not just about charts and numbers; it's a strategic fusion of data, AI, and design to create powerful, engaging, and insightful content.
Data storytelling is the process of translating complex data into a structured narrative that is easy to understand, engaging, and actionable. According to Catherine Cote, data storytelling combines three core elements.
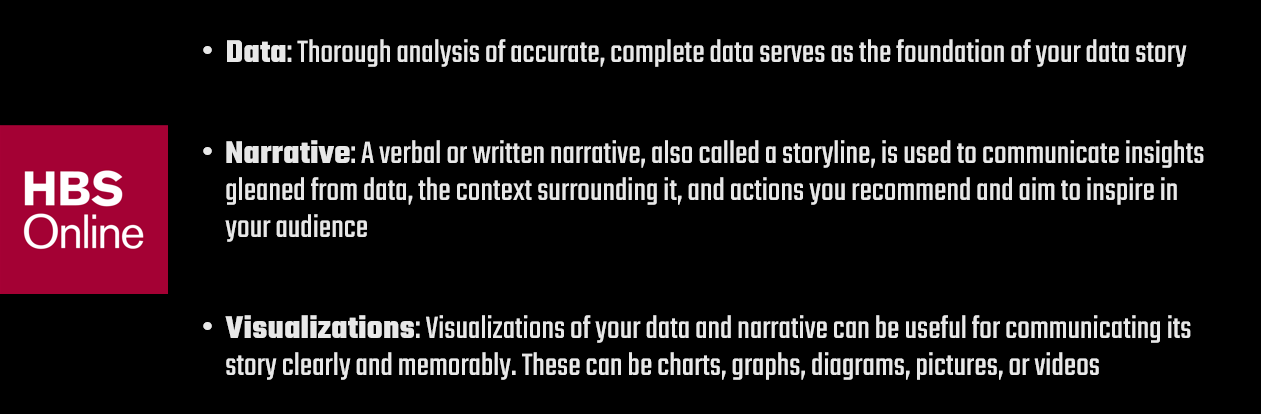
When done right, data storytelling informs, persuades, and inspires action. It’s used across industries—from journalism to marketing to sports analytics—helping businesses and creators make data more accessible and compelling.
What is the Opportunity?
Data storytelling is a significant opportunity within the creator economy. For example, in 2024 the creator economy was worth $156.4B, and by 2030 it'll be worth $528.4B. Further, there are 50M content creators worldwide, and this number grows every day.
Today, more than ever, we're seeing independent content creators join the creator economy. Storytelling is becoming more decentralized with social platforms providing new opportunities within this new and growing economy. For example, one of the domains we focus on is sports, and with just YouTube, there are massive engagement numbers.
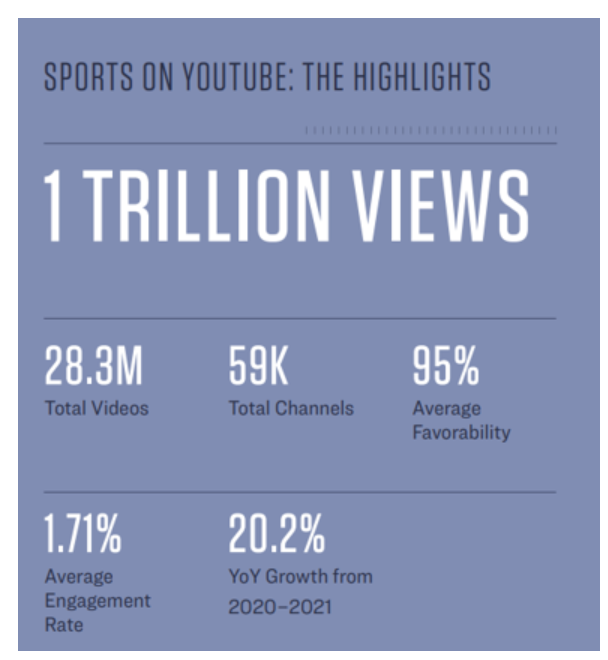
The creator economy is changing what it means to be an independent creator. And data storytelling is one of the ways in which creators can meaningfully engage within the creator economy. And the opportunity for sports-minded content creators is significant and growing. And while we'll focus on sports data storytelling in this series, we see opportunities across many domains such as sports, news, conservation, politics, and entertainment.
The Role of AI in Data Storytelling
AI has revolutionized data storytelling, making it more efficient to research, analyze data, detect patterns, and optimize content-generation efforts. In our projects, we leverage AI in several areas that have proved invaluable. For example, we've listed out a sampling of the technology we use in our projects – across the areas of research, data analysis and content – in the following diagram.
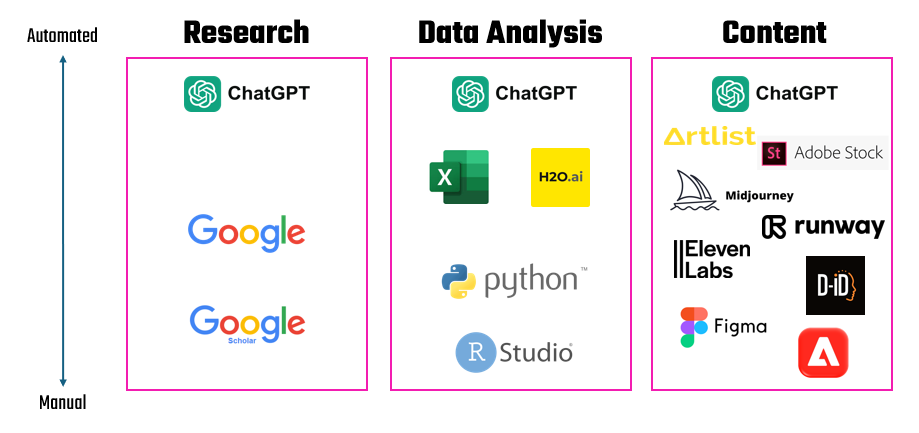
When we leverage AI, there some instances where what we do is very automated, such as a search query, prompt or the click of a button on an AI-enabled website. In other instances, we may have to write code (to implement AI programs), draft scripts, source and transform data (as input into our data analyses), or create base visual images to use as input into the AI or machine learning algorithms we may be building. Of note is that we also heavily rely on non-AI resources, such as books and websites, which we use throughout our project lifecycle. And for some of our projects, we use designers and professional voice actors to help with content production.
Let's explore each of the areas in the above diagram.
Research
Our initial step in a project is to take notes, understand the goals and objectives of the project, enumerate key questions we're trying to address, and sketch out an initial approach. During this phase, we'll likely use ChatGPT, search engines and scholarly papers to learn more about a specific topic. We keep a running OneNote for each project, which we use to capture key links, notes or even code snippets we find that might be helpful later on to test out concepts. We also use Miro for brainstorming, building mind maps and process flows, and designing wireframes.
Data Analysis
During the data analysis phase, we use AI as a smart companion in our analytical research and modeling (e.g., ChatGPT) and as a way to do quick exploratory analyses (e.g., using Excel's built-in AI data analysis features). Given we do a lot of one-off projects, it means we have to learn on the fly and do research on specific topics.
After we have done some initial analyses, we'll then code up more specific analyses in Python or R. We tend to use Python for more data management operations and R/RStudio for data science modeling. If we need to implement using a specific enterprise framework, then we'll do that – which may mean using more Python end to end.
Note that we don't substitute AI tools for our own analysis – e.g., we may do research using ChatGPT, but we still build our own models in Python and R based off of our own data engineering. Thus, AI is a complement to our machine learning skills and knowledge and is a force multiplier to get more done in a shorter period of time (and shift productivity to higher-value work).
Content
We use a broader range of AI services and websites in this area – especially if we don't have a designer for a project. For example, you can generate draft content outlines, which you can then tailor with your own knowledge and experience; you can create images and short videos; you can source stock footage, images and sounds; you can create amazing images through prompt engineering; and you can leverage templates to bring it all together.
What's key here is don't overdo the use of AI in generating your content. It may take you some time to find a good balance in your content, so take the time to experiment. Also, you'll find that there are places where you will want to continue to use a designer and places where AI can help more. For example, we leverage AI more in long-form articles and use a designer for our socials, infographics and videos. Experimenting with how to generate and assemble content helped us think more productively about AI and to understand the best places to use it.
Lastly, AI-driven design tools can generate data visualizations dynamically, choosing the best type of chart or graph based on the dataset. Tools like Datawrapper, Flourish, and even AI-assisted design software like Canva and Figma help creators develop more engaging, visually compelling stories with less manual effort.
What is Data Storytelling for Sports?
Sports data storytelling differs from traditional data storytelling because it deals with real-time, highly engaging, and emotionally-charged content. Fans, analysts, and teams all consume sports data differently, requiring stories that not only inform but also entertain and provoke discussion.
Some of the main distinctions include:
- Real-Time Data. Sports analytics often require live data updates during games, which means data stories must be dynamic and adaptive.
- Emotional Engagement. Sports fans are passionate; storytelling must tap into rivalries, records, and historical comparisons to resonate.
- Competitive Analysis. Unlike business or finance, where trends might evolve over months or years, sports data storytelling needs to break down performances on a game-by-game or season-by-season basis.
- Player & Team Performance Metrics. Sports storytelling focuses heavily on specific stats like expected goals (xG), possession percentages, shot maps, and player efficiency ratings, all of which require contextual explanations.
- Fan-Friendly Visualization. Sports data should be easily digestible, favoring interactive dashboards, highlight-infused graphics, and visual comparisons between players, teams, and historical performances.
Key Considerations for Sports Data Storytelling
If you’re crafting a compelling sports data narrative, here are some factors to keep in mind.
Know Your Audience
Are you targeting casual fans or hardcore analytics junkies? Casual fans need simpler metrics like Goals per Game, while data-savvy readers want advanced stats like Corsi, PDO, or WAR (Wins Above Replacement). Tailor your depth of analysis accordingly.
Choose the Right Metrics
Not all stats are created equal. Pick stats that tell a clear story. For example, instead of showing Total Shots, a better measure might be Expected Goals (xG), which accounts for shot quality. In hockey, comparing Corsi vs. Fenwick can reveal different aspects of puck control.
Context is Everything
A number alone doesn’t tell a story—context does. For example, saying a player has a 70% save percentage might seem bad, but if they faced 50 shots per game, the context changes. Always compare against league averages, historical trends, or similar player/team performances.
Include Predictive Insights
AI and machine learning techniques can help you analyze trends and predict future performance. For example, AI models can estimate how many goals a player will likely score based on past shot patterns, teammates, and defensive matchups. AI-generated insights can fuel pre-game storylines, betting analysis, and team scouting reports. They also make a sports data story more interesting by proposing a future event.
Emphasize Rivalries and Comparisons
Sports fans love comparisons—past vs. present, team vs. team, player vs. player. For example, a side-by-side chart comparing Connor McDavid with Wayne Gretzky can spark debate and engagement. Use historical data to frame modern performances in a broader context and compare vintage with the modern.
Use Engaging Visuals to Complement Statistics
Fans don’t want to sift through raw data—use compelling visuals like:
- Shot heatmaps (showing where a player takes the most shots)
- Possession flowcharts (tracking team dominance)
- Career trajectory curves (comparing players over time)
- Game trend graphs (momentum shifts in a match)
You can use different tools to build out these visuals, for example, Flourish, DataWrapper, Tableau or Python. You can also look for ways to animate them more dynamically within your data story using JavaScript, D3 or Plotly.
Don't Just Recap; Tell a Story
Instead of just listing stats, create a narrative arc, for example:
- Create a question that frames the data story, e.g., Is this the best season of McDavid’s career?
- Use key metrics in your data analysis to support the argument.
- Provide easy-to-digest charts and visualizations.
- Offer insights, projections, or comparisons to history.
Make It Interactive
Sports fans love to explore data themselves, so where possible make the data story interactive. Interactive visualizations allow a user to:
- Compare player stats over different seasons.
- See how a team’s power-play percentage ranks historically.
- Track real-time game analytics.
Tools like Power BI, Tableau and Flourish can make data exploration easy.
Example Data Story: When do NHL Superstars Peak?
To make this series more practical, we're going to introduce and progressively build out a data story. By doing this, we'll cover the data analysis, translate that analysis to a narrative, find the hook, apply design principles to the narrative, leverage AI, and finally produce the data story. We'll also refer to resources and templates that we're building and releasing for creators in the space and showcase demo projects that show you the potential of what you can create.
Most data stories come down to a simple question, and in our data story example the question at the heart of this sample data story is: When do NHL superstars peak? NHL superstars are the top of the league in terms of performance, but even the best athletes follow a natural age curve.
In each newsletter in this series, we'll walk through the specific steps you would take to build out the data story. The goal of the data story will be for you to take the sample data and follow along to build out your own sample data story. You can choose to build the same story as is in the course, or you can take the data and choose to do something else with it.
Whatever path you choose, our goal will be to have you create a better data story than us.
Summary
This was the first newsletter in our email course on Data, AI & Design: Data Storytelling in Sports. In this edition, we:
- Introduced you to Data Storytelling
- Summarized how you might leverage AI in your data
- Discussed what is unique to Data Storytelling in Sports
- Introduced you to the case study example we'll use throughout the course
In our next lesson, we'll focus on exploring the data to find our narrative for the data story.
Subscribe to our newsletter to get the latest and greatest content on all things data storytelling for journalists and content creators!